#1 Where are you on the AI-readiness scale?
Your granddaughter is using chatGPT in their kindergarten. It was you who taught her, and nobody can distinguish your last trip photos from the freshly generated ones that you post on your feed.
You've read all the articles about AI in Forbes and the Economist, and now you see the way to implement this new-gen tech in all the business processes of your company...
...but still, only 23.51% of your colleagues know the difference between LLM and LVMH, don't they? Welcome to the FOMO club, guys :)
So, it seems that technology is mature enough. You too. But what about your company?
For the last few years I have been involved in very different projects, among them were: building the intelligent analyst assistant (we got to properly test out Claude v2 on Bedrock), exploring tons of IoT data coming from cooking devices, looking at the open data related to long COVID, improving omnichannel recommender system, building documents compliance verification; I devoted some time to predictive maintenance, worked on automating visual inspection in Oil&Gas facilities, and ... and I stop bragging here 🙂 I love it, it is fun!
And the concept which was often silently present independently of the domain of the project is how actually ready the company and the process C-level wants to improve were for the AI-based solution.
So let us dive into it. This post is a bit longer, so please welcome - the landscape for it:
- Definitions
- How AI readiness is measured on a country level?
- Where is your company on the scale?
- Where are you personally?
So, definitions.
Readiness is generally framed as an ability to get value and benefits from tools and technologies in our case from AI. Our friend of the last months - if you don’t get that I'm referring to LLMs, we might have some trouble getting along, if you're asking "LLM? What the heck is it?", we both might guess where exactly you stand on the AI-readiness scale before reaching the end of the blog. And it is OK to be there. So, this friend defines it in the following way:
AI readiness refers to the state of preparedness or capability of an organization, society, or individual to effectively adopt, implement, and leverage artificial intelligence (AI) technologies for various purposes. It involves having the necessary infrastructure, resources, skills, and mindset to embrace AI in a way that aligns with the organization's goals and objectives.
It might sound like the usual generic stuff and it is generic, even more, it is boring but often it is that heavy lifting underneath sugary coated promises to empower your business in a few clicks with a simple API.
So, countries.
Oxford Insights has been publishing Government AI readiness index for 5 years, let us look deeper into the ranking and methodology. For the 181 countries included in the index evaluates: how ready is the government to implement AI in the delivery of public services to their citizens? Index measures 39 indicators across 10 dimensions, and 3 pillars: Government, Technology Sector, and Data & Infrastructure.
Summary:
Global Leaders: USA tops the index, but Singapore leads in two out of three pillars.
Regional shakeup: Western European countries make up fewer than half of the top 10 countries for the first time as three East Asian countries achieve top 10 positions.
Government Pillar: AI strategy work is dominated by middle-income countries.
Technology Sector Pillar: AI skills are global, with future developers emerging in a diverse set of countries.
Data & Infrastructure Pillar: Changes to the Data & Infrastructure Pillar expose which governments are taking an active role in data availability and puts three East Asian Countries at the top of the dimension.
Most countries are struggling with the technology sector, namely with maturity, innovation capacity, and human capital. Let us check some trends inside these pillars specifically. The indicators inside them are:
- Maturity:
- Number of AI Unicorns
- Number of non-AI Unicorns
- Value of trade in ICT services (per capita)
- Value of trade in ICT goods (per capita)
- Computer software spending
- Innovation Capacity:
- Business administrative requirements
- VC availability
- R&D Spending
- Company investment in emerging technology
- AI research papers (log transformation)
- Human Capital:
- Graduates in STEM or computer science
- Github Activity
- Female STEM Graduates
- Quality of Engineering and Technology Higher Education
- Digital skills
Below are a few top insights based on these indicators. Graphs and link to the open dataset can be found below in the appendix.
- The concentration of R&D spending and company investment in a few countries.
- The concentration of AI and non-AI unicorns in a limited number of countries.
- The wide variation in the quality of engineering and technology education.
Not a big surprise, right? These insights are especially clear from a system analysis point of view. Let me cite a nice book on system thinking here:
According to the competitive exclusion principle if a reinforcement feedback loop rewards the winner of a competition with the means to win further competitions, the result will be the elimination of all but a few competitors
Or to say it in a simpler way - "The rich get richer and the poor get poorer". Surely, the underlying causal relationships are more complex but still without interventions (and kudos to Open Source!) that is what we get.
So, companies.
It is pretty obvious that similar metrics could and should be used for companies. A few assessments are available, I particularly liked the approach by AI Singapore (AISG) with the summary below.
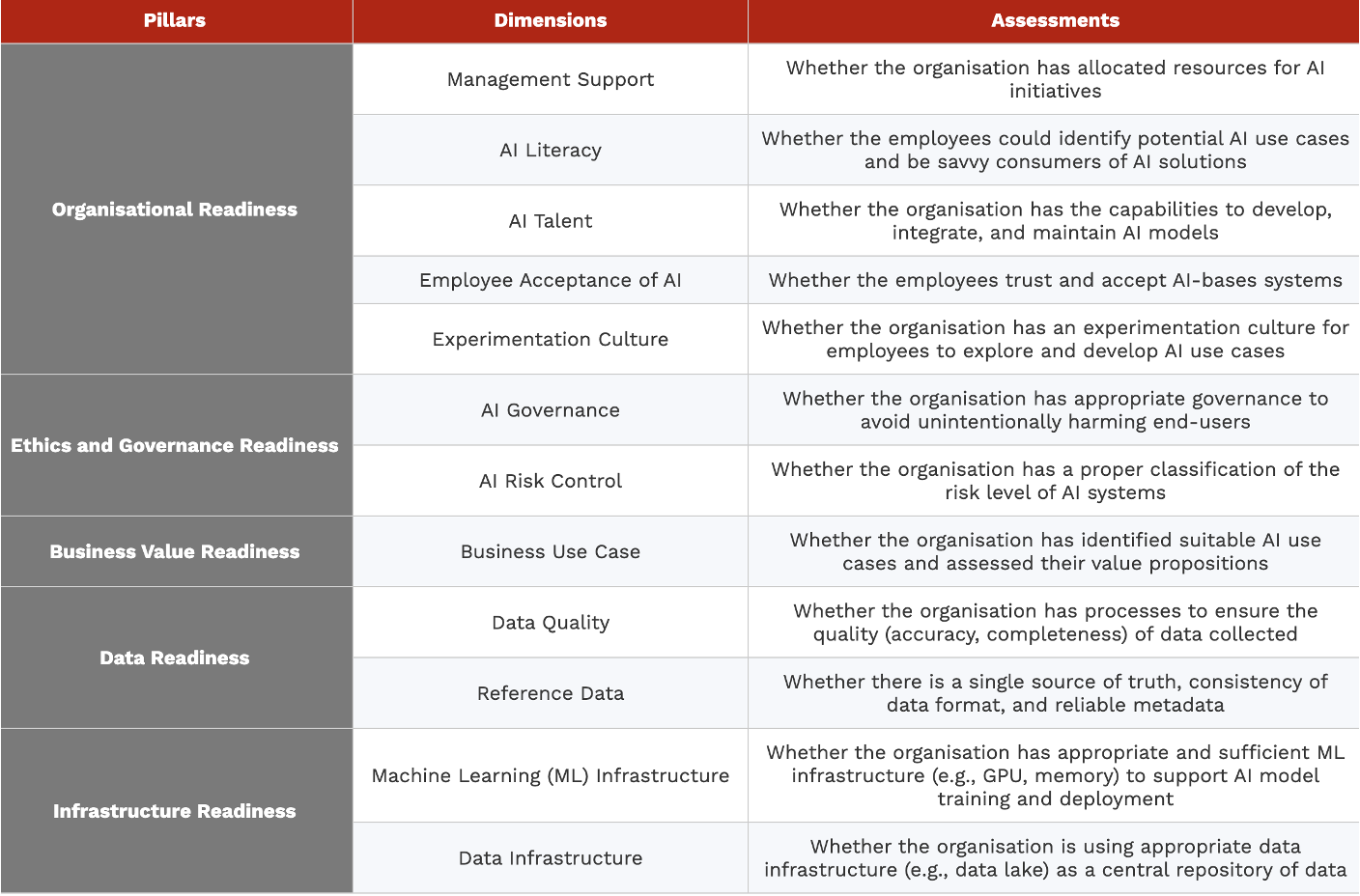
If you scan the dimensions, do not be fooled by high-level descriptions, just imagine how ready the company actually could be in case a few of the dimensions are very low. How far the initiative could go without management support and/or not-developed infrastructure? I use this as a short reference before any project to predict if the AI proof of concept (PoC) will be successful and what high-level risks are already visible.
I hope you're still here and we have not reached the end of your attention span, hold on, a few more schemas and you will be ready to look at the world differently! (I'm being ironic, remember?)
Below is also a very useful reference for checking how mature any process is. For instance, if in your company the Software Development Life Cycle (SDLC) processes are not the optimal way, how could you productively and at scale add GenAI to automate some tasks? Or something even simpler, once we were asked to build a fault prediction system but there were literally only hand-written piles of documents, no one looked at them for years, and no process around. Predicting faults PoC? Really?
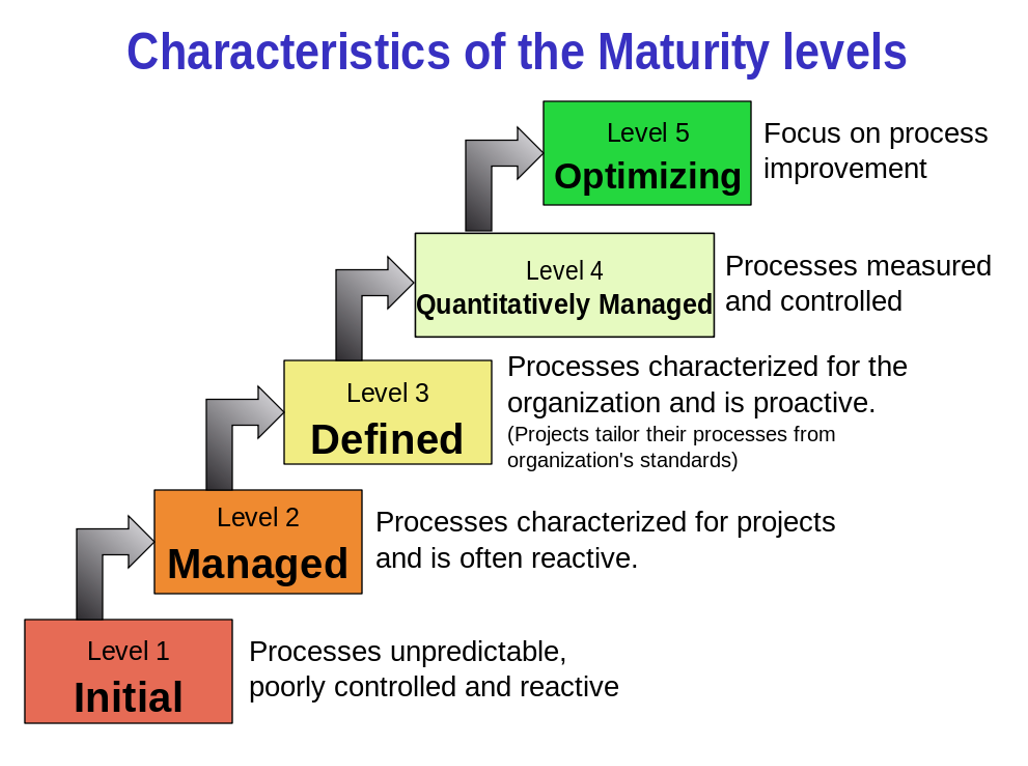
To sum up, the process you want to enhance/automate/add some AI magic to might be just not in the right state of mind and there are even more high-level risks smart people from Singapore framed together in the picture above. Remember Singapore is the country that leads in two out of three pillars the first AI index described. Coincidence? Don’t think so. (Still ironic)
Wrapping up this part of the story. Where are you on the AI-readiness scale?
For instance, what would be your thoughts if you knew that your doctor was using an LLM-based health assistant to help with your diagnosis? And if that was your child’s doctor? Ok, something a bit simpler, what are your associations when you see 'AI-powered' on a website?
Let me add more specific questions and I left you for the self-evaluation process.
- What is your understanding of fundamental AI principles, such as machine learning, neural networks, and natural language processing?
- Determine how comfortable you are in handling data, interpreting data insights, and understanding data-driven decisions.
- Examine your awareness of data security best practices and your ability to protect your personal data when using AI applications.
- How many prompt engineering techniques do you know?
- What routine tasks you’d be willing to automate? Have you searched for the special tools for that?
- Would you like to know if any AI/ML solution is used by others to provide services for you? Why?
What about the happy ending? Not really, the capabilities GenAI opened along with overall low data/AI literacy is an interesting combo, to say the least. So, keep on watching and learning, and see you soon.
Appendix
Open Dataset for Government AI Readiness 2022
A few histograms for the technology sector dimensions.
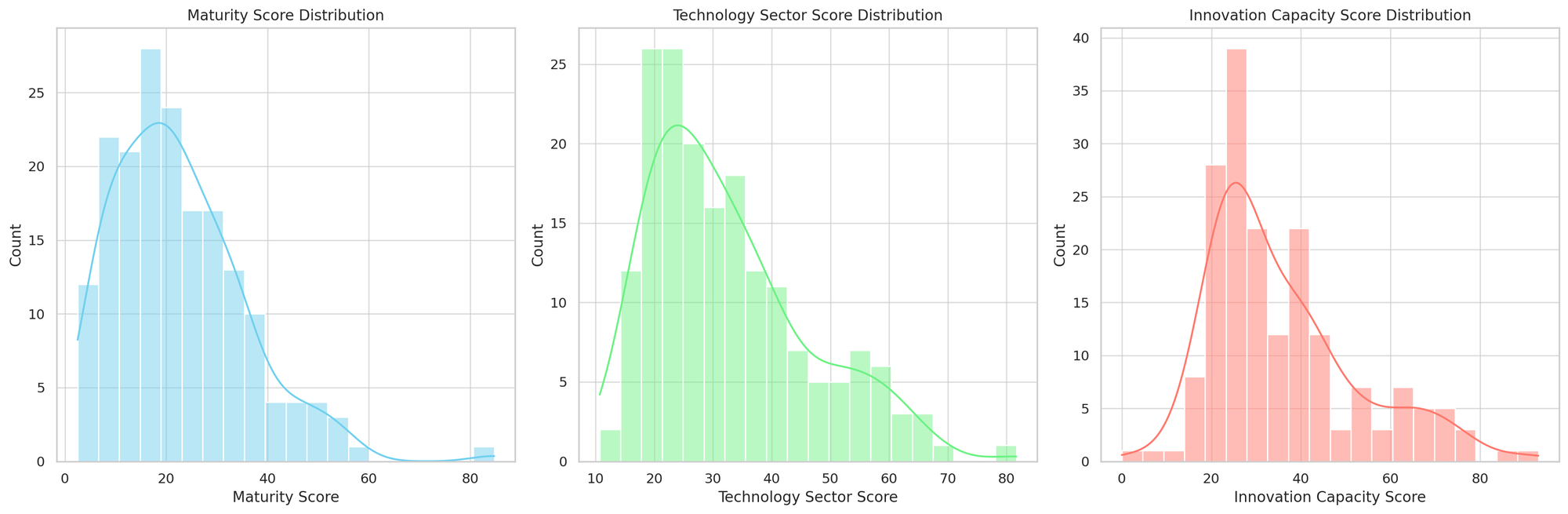
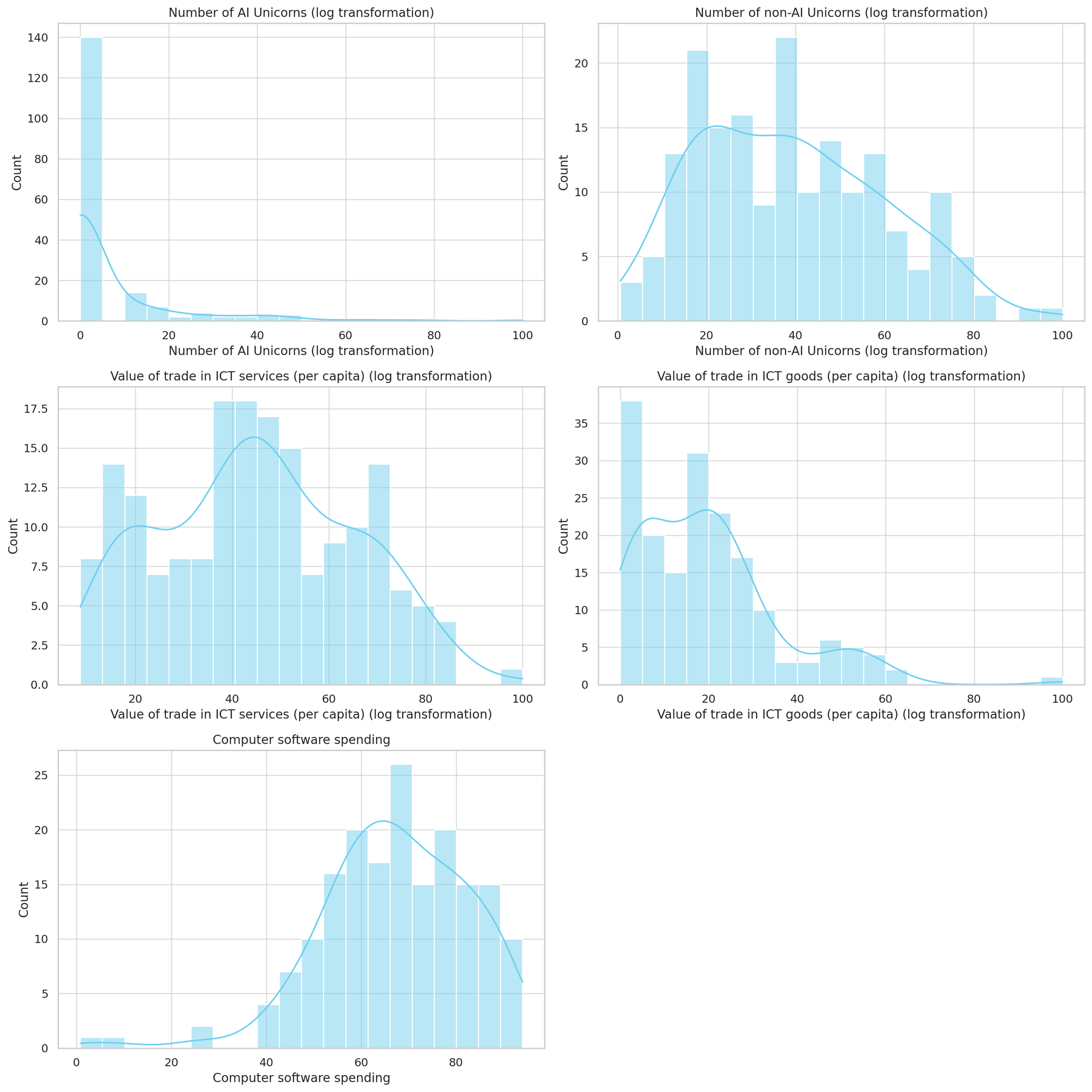
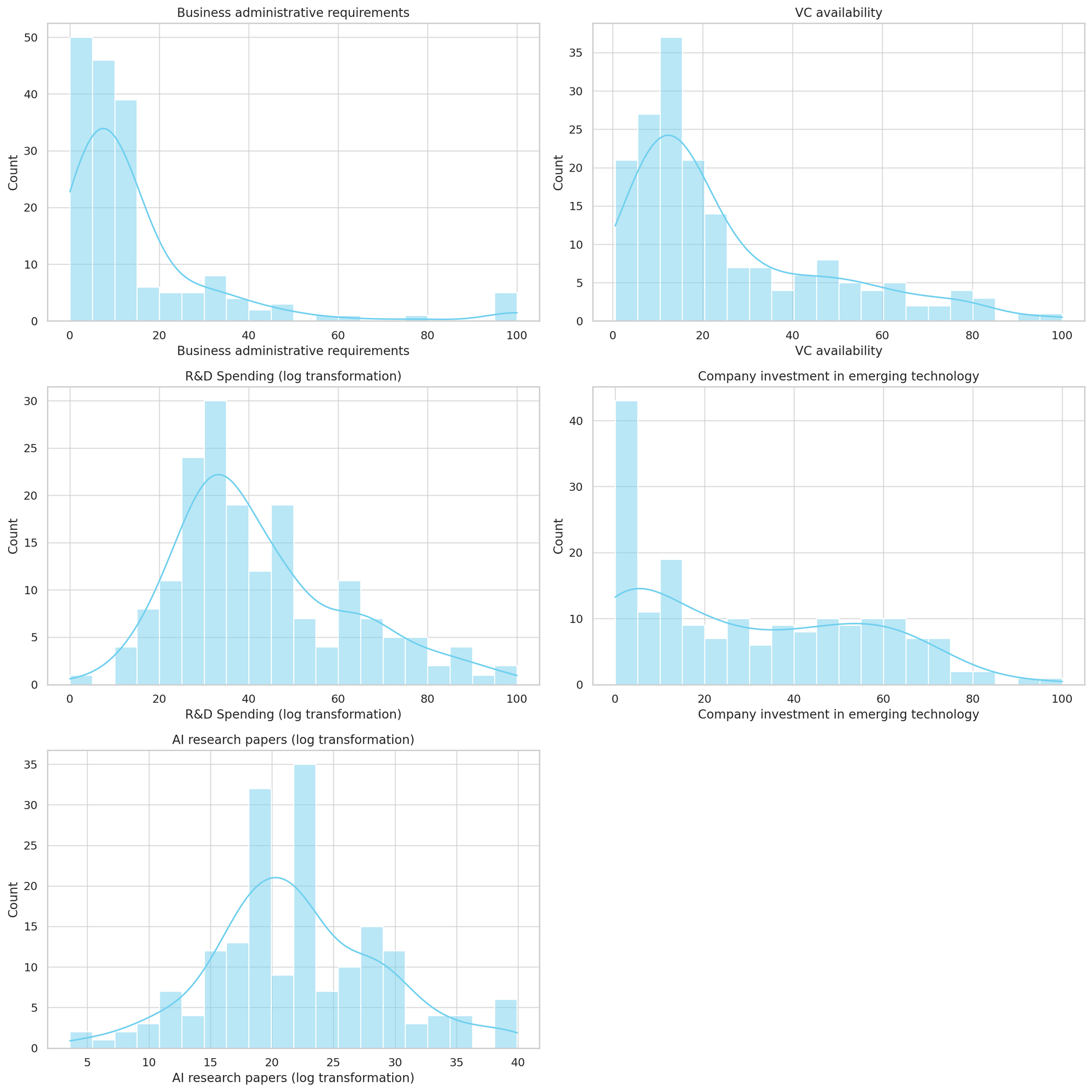